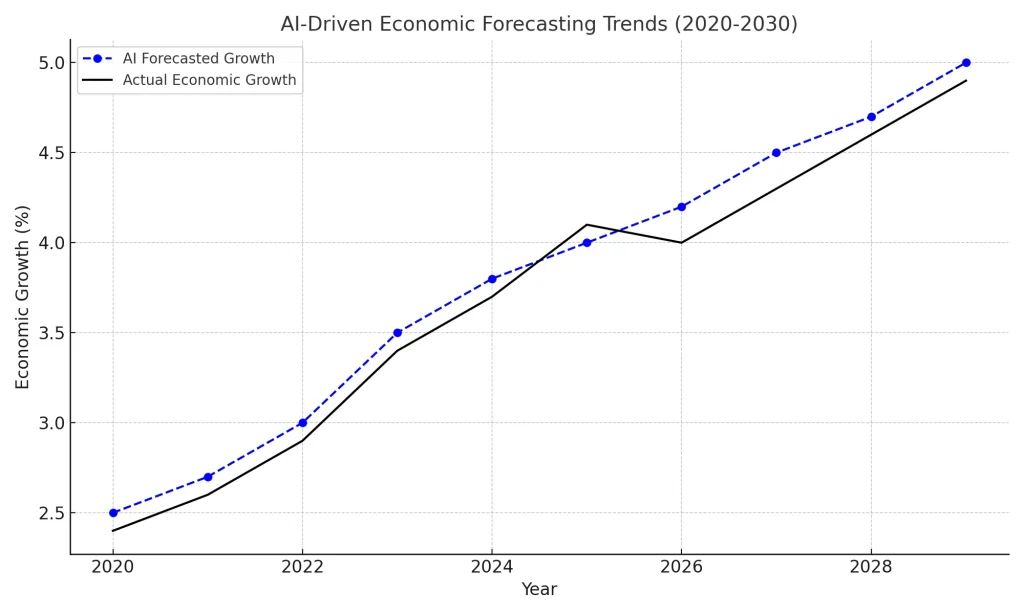
Artificial intelligence (AI) is transforming the way economic forecasting is conducted, revolutionizing how we predict and interpret financial trends. AI technologies, like machine learning and deep learning, automate complex forecasting tasks, offering faster and more accurate insights. As the global economy becomes increasingly complex, understanding the role of AI in economic forecasting is crucial for professionals in financial planning, market research, and investment decision-making. This review explores how AI is changing the game in economic forecasting and what it means for the future of financial analysis.
Overview of AI in Economic Forecasting
AI is fundamentally reshaping economic forecasting by automating tasks that were once manual and time-consuming. Traditional forecasting methods relied heavily on human analysis of data sets, but AI can now analyze massive amounts of economic data in real-time. Machine learning algorithms, for example, can identify patterns in GDP trends, consumer spending, and stock market behaviors that would be difficult for humans to spot. With features like real-time data analysis and pattern recognition, AI enables more precise predictions, especially in sectors that require quick adaptation to changing market conditions. This shift allows economists and analysts to base their predictions on more comprehensive data sets, improving overall accuracy.
Pros & Cons of AI in Economic Forecasting
AI offers a wide range of benefits in economic forecasting, including enhanced accuracy and speed. AI models can process vast amounts of complex, non-linear data that would otherwise overwhelm human analysts. This improves the ability to forecast trends in areas such as inflation rates, stock market fluctuations, and even global financial crises. Additionally, AI can automate financial tasks like data collection and initial analysis, freeing up human analysts for more strategic decision-making.
However, AI in economic forecasting is not without its limitations. There’s a significant risk of over-reliance on AI models, which may produce inaccurate results if fed low-quality data. Furthermore, AI models can struggle with rare, unexpected events, like pandemics or geopolitical shocks, which are difficult to predict. Ethical concerns also arise, particularly around transparency—many AI models operate as “black boxes,” making it hard to understand how decisions are made. Below is a table summarizing the key advantages and disadvantages:
Pros | Cons |
---|---|
Higher accuracy in trend prediction | High dependence on data quality |
Automation of financial analysis tasks | Lack of transparency in decision-making |
Quick processing of large datasets | AI models struggle with rare, unforeseen events |
In-Depth Analysis of AI in Economic Forecasting
AI-Powered Tools and Techniques
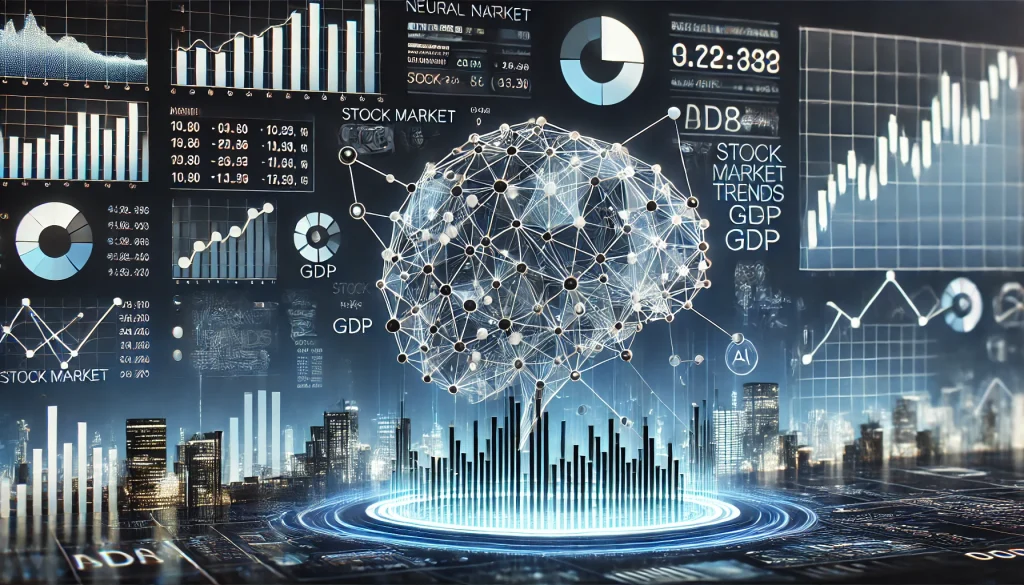
Various Artificial Intelligence tools are employed in economic forecasting, from neural networks to sentiment analysis. Neural networks can predict economic trends by learning from historical data and adjusting to new inputs. Sentiment analysis, on the other hand, analyzes social media posts and financial news to gauge public sentiment, which can predict market behavior. Machine learning models like random forests and support vector machines also help analyze GDP data, market volatility, and consumer behavior, providing more nuanced insights into economic forecasts.
Performance and Accuracy
Artificial Intelligence generally outperforms traditional economic forecasting models in several areas, especially in handling large, complex data sets. Studies have shown that AI-based models can provide more accurate predictions for stock markets and inflation rates by processing unstructured data such as social media sentiment and online news articles. For example, AI-driven models have been able to predict market downturns by identifying early warning signals in real-time, something that traditional models often miss.
Usability and Adoption
While AI-powered forecasting tools are highly effective, their usability can be a challenge for some. Financial institutions and analysts may struggle to adopt these tools due to the technical knowledge required to operate them. The skills gap in machine learning and AI remains a barrier for many economists and analysts. However, as AI tools become more user-friendly, their adoption is expected to grow, particularly among large financial firms and institutions.
Comparison of AI vs. Traditional Forecasting Methods
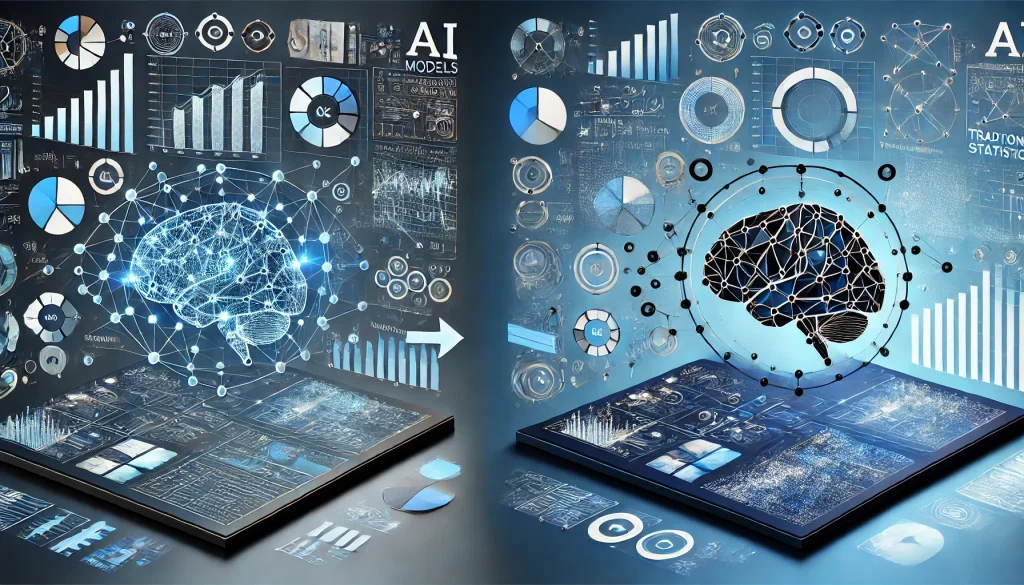
When comparing AI-driven economic forecasting with traditional statistical methods, several key differences emerge, primarily in how they process and interpret data. Traditional models, such as ARIMA and regression analysis, rely on structured data and predefined relationships between variables. These models are effective in stable environments where historical patterns are consistent, but they often struggle with non-linear, complex datasets. In contrast, AI models—such as neural networks and machine learning algorithms—can analyze vast amounts of unstructured data, including real-time inputs like social media sentiment and online news, which traditional methods cannot process.
Another significant difference is in speed and flexibility. AI models can analyze large datasets much faster than traditional methods, allowing for quicker decision-making. While both aim to predict economic trends, AI’s adaptability and ability to uncover hidden patterns provide more dynamic and accurate forecasts, particularly in volatile markets. However, traditional models are more transparent, making their results easier for economists to interpret and explain, whereas AI models often function as “black boxes,” producing outputs without clear explanations of how the predictions were derived.
Ultimately, AI complements traditional methods rather than completely replacing them. In many cases, combining both approaches—leveraging the flexibility of AI and the reliability of statistical models—can yield the best results.
Challenges and Limitations of AI in Economic Forecasting
Despite its advantages, AI faces several challenges in economic forecasting. One of the most significant issues is data quality. AI models require vast amounts of accurate, high-quality data to function effectively. If the data is incomplete, outdated, or biased, the predictions generated by AI may be flawed. This makes data collection and curation critical for ensuring the reliability of AI-driven forecasts.
Another limitation is the interpretability of AI models. Many AI techniques, such as deep learning, operate in a way that makes it difficult for human analysts to understand how decisions are being made. This lack of transparency can create a trust gap between AI models and the economists or financial institutions that rely on them, especially when the stakes are high, such as in investment decisions or policymaking.
Ethical concerns also arise with the use of AI in economic forecasting. Bias can easily be introduced into AI models if the data they are trained on reflects historical inequalities or prejudices. For example, an AI model that uses biased economic data might produce forecasts that disproportionately affect underrepresented groups or lead to skewed policy recommendations. Additionally, there are concerns about over-reliance on AI. While AI can offer more precise predictions, unforeseen events—such as natural disasters or pandemics—can throw off even the most sophisticated models, as they rely heavily on past data to make future predictions.
To address these challenges, AI developers and economists need to collaborate on creating more transparent models and establishing ethical guidelines to mitigate bias, while continuing to improve the quality and accessibility of data for economic forecasting.
Conclusion
AI is revolutionizing economic forecasting by increasing the accuracy and speed of predictions, particularly in fast-moving financial sectors like stock markets and inflation trends. As the technology continues to evolve, tools like neural networks, sentiment analysis, and machine learning models are becoming more sophisticated, allowing economists to forecast with greater precision. However, challenges remain, especially concerning data quality, interpretability, and ethical concerns. Looking ahead, advancements like quantum computing and more transparent AI algorithms may further enhance AI’s role in economic forecasting. Financial institutions and analysts should leverage AI tools to stay competitive, but they must also remain cautious about their limitations.
FAQs
How does AI improve the accuracy of economic forecasting?
AI enhances accuracy by processing vast amounts of complex data in real time, identifying patterns, and making predictions that are often more precise than traditional models.
What are the limitations of AI in economic forecasting?
AI’s limitations include dependence on high-quality data, challenges in predicting rare or unforeseen events, and issues related to the interpretability of AI models by non-experts.
Can AI fully replace traditional forecasting methods in economics?
While AI provides deeper insights and faster processing, it complements rather than fully replaces traditional methods, as human expertise is still needed to interpret results and manage unexpected variables.
Resources
- ResearchGate. The Impact of AI on Economic Forecasting and Policy-Making
- Wiley Online Library. Artificial Intelligence in Economic Forecasting: A Review
- Medium. AI and Economic Forecasting
- McKinsey & Company. AI-Driven Operations Forecasting in Data-Light Environments
- MDPI. Artificial Intelligence Techniques in Economic Forecasting